QuantumSi Prospectus Review
This post originally appeared on the substack.
I previously looked at QuantumSi’s protein sequencing approach back in September. But recently someone forwarded me their prospectus. Having recently reviewed Nautilus it seems like a good idea of revisit QuantumSi. In this post I provide an update based on my previous thoughts but you may want to refer to that post for details from their patents.
Technology
The basic process QuantumSi use to sequence proteins can be briefly described as follows:
- Fragment proteins into short peptides, and isolate in wells.
- Attach a label to the terminal amino acid, and detect the label.
- Remove a single terminal amino acid.
- Go to step 2 to identify the next amino acid.
At a high level is not unlike single molecule sequencing-by-synthesis, in that monomers are detected sequentially. The difference here being that rather than incorporating monomers, in this approach they are cleaved. QuantumSi appear to fragment the proteins prior to sequencing. I assume this is to avoid secondary structure issues. But it does mean they are getting fragmented sequences rather than an end-to-end sequence for the entire protein.
When I reviewed their patents, it was reasonably clear that you’d be unlikely to get an accurate protein sequence. It’s more likely to be a fingerprint. This means that rather than being able to call a “Y”, you’d likely be able to say this amino acid is one of “Y,W or F”.
The Prospectus suggests that can resolve these ambiguities by looking at transient binding characteristics. The “affinity reagents” they use don’t bind and stay attached. Rather they have on/off binding. So you’ll see them attach, generate a signal, then detach, then another one bind etc. Ideally a reagent that binds to “Y,W or F” might bind more strongly to one (e.g. Y) than another (e.g. W) and you can use that information to infer the amino acid type.
As mentioned in my previous post, they use fluorescent lifetime determine which affinity reagent is bound. So for every detection event they have two pieces of information, the affinity reagent type (from fluorescence lifetime, and intensity) and the binding kinetics (from the on/off rate). They call this 3 dimensional data (fluorescence life time, intensity, and kinetics).
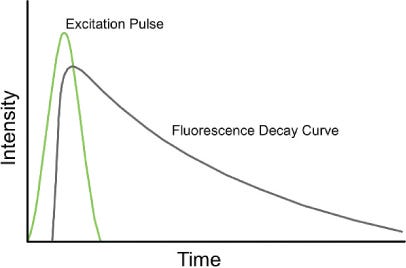
The nice thing about this is that while you will need various reagent types, you don’t need a complex fluidic system and you are observing, and classifying them in real time.
However, I’ve not seen anything that suggests the classification works well enough to give the full sequence. And they state that this “will ultimately enable us to cover all 20 amino acids”. Suggesting that they currently can’t.
Overall, the above approach is in line with my previous speculation based on their patents.
Chips
Like Ion Torrent, they make a big deal out of using semiconductor fabrication for their sensor: “similar to the camera in a mobile phone, our chip is produced in standard semiconductor foundries”. I generally take issue with this argument. Semiconductor fabrication is great. But if you can’t reuse the sensors it’s more like buying an expensive camera, taking one picture, then throwing the camera in the trash.
This isn’t to say that semiconductor sensing isn’t interesting… but there are other issues that need to be considered. They also talk about Moore’s law, suggesting that if “Moore’s Law remains accurate, we believe that single molecule proteomics…will allow our technology to run massively parallel measurements”. Aside from Moore’s law clearly being in trouble, this doesn’t make much sense, as there are other physical limits involved here.
From various public images, I’d guess the chip is ~15 to 20mm². PacBio chips (which use a very similar approach) have ~8M wells on a chip that appears to be roughly the same size. I didn’t see an explicit statement on read count, other than “parallel sequencing across millions of independent chambers”. But my best guess would be in the 10M range.
This puts them at the low end of throughput as compared with Nautilus and other next-gen proteomics approaches.
Product
The product has 3 components. A sample prep box (Carbon) the sequencing instrument (Platinum) and a Cloud based analysis service. Unlike Nautilus they suggest that primary data analysis happens on instrument. The instruments combined pricing is supposed to be in the $50,000 range, which is relatively cheap.
Commercial Stuff
QuantumSi say they have already initiated their early access program, but I’ve not heard of anyone else talking about this publicly. They are aiming for a commercial launch in 2022. And say that their addressable market is $21 billion. This breaks down as follows:
Of this, I think the true addressable market is closer to the $5B legacy proteomics segment. It doesn’t seem realistic to use the proposed approach for health care/diagnostics in its current form. Partly because the per-sample COGS is likely pretty high, and partly because for these applications you may want a higher throughput instrument.
They also suggest that in the future they will release lower cost instrument for at home testing:
Conclusion
QuantumSi’s approach is closer to sequencing than Nautilus, but I suspect the platform will still not give a true amino acid sequence when initially released. For the reasons highlighted in my previous post protein sequencing is just much much harder than DNA sequencing. So, like Nautilus what they’re developing may be more of a protein fingerprinting device, where traces are compared against a database of known proteins.
This begs the question: what’s the value in a relatively low throughput protein fingerprinting instrument? Where exactly the throughput spec needs to be set to be useful, particularly for diagnostic applications isn’t clear to me. But 10 million reads would certainly seem to be on the low end. I’ll try and address this in a future post.