Nautilus Biotechnology
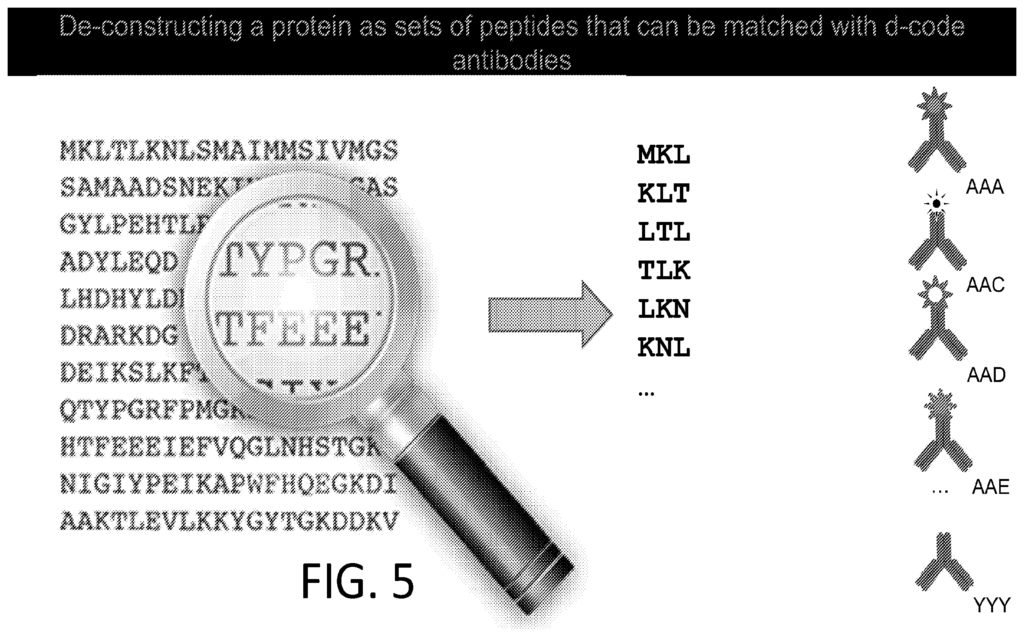
This post previously appeared on my substack.
Company Background
Nautilus (originally Ignite Biosciences) was founded in 2016 by Parag Mallick and Sujal Patel. Sujal was previosly CEO of storage company Isilon. Isilon storage gained huge popularity in genomics for storage next-gen sequencing data. They had deal with Illumina at one point, and were probably the easiest way of getting scalable storage up and running. More recently Isilon’s popularity in genomics seems to have wained, with users switching over to cloud based solutions.
A youtube interview with Sujal covers Nautilus’ background and how Sujal got involved in Biotechnology coming from a tech background. Parag, like many others was using Isilon’s platform for genomic applications. In his interviews Sujal draws parallels between Nautilus’ proteomics platform and the explosive growth of next-gen DNA sequencing.
However, Sujal also positions Nautilus as most appropriate for Pharma applications. This is very different than next-gen DNA sequencing where Pharma was not an early driver of growth (and still isn’t).
They raised a $76M series B in May 2020. And like seemingly everybody else, are doing a SPAC.
Technology
Nautilus Biotechnology is building a high throughput single molecule protein fingerprinting platform. There are a few other companies doing this (Encodia, QuantumSi, Dreampore, Erisyon (disclosure, I’ve worked with Erisyon in the past, but hold no equity)).
Looking over their patents, there seem to be 3 areas of innovation:
- A method for arraying single proteins on a surface.
- A method for identifying/fingerprinting proteins.
- A method for developing libraries of affinity reagents for use in fingerprinting.
I’ve cover each of these in turn below and then review the complete approach.
Arraying Single Proteins
If you randomly stick proteins (or anything else) to a surface there will be some probability that two of more proteins will be right next to each other. If that happens you wont be able to resolve the single proteins and a mixed, unusable, signal will be generated.
So, random attachment limits throughput. Many platforms run into this problem, in Solexa/Illumina sequencing on the Genome Analyzer 40% of reads were from “mixed” clusters and were discarded. On Oxford Nanopore’s device you have bilayers/wells with multiple pores which are not easily usable. In general such approaches are “Poisson limited” in a well based system this means 37% of wells will have single occupancy.
Most platforms attempt to solve this at some point. Illumina introduced patterned flowcells and ExAmp. Genia have worked on a pore insertion approach, to ensure single occupancy.
But in general, it’s not something that makes or breaks a platform. It only limits throughput, doesn’t effect data quality. So it’s usually addressed in a second generation product.
Nautilus however have been working on this issue for proteomics. Why they are focusing on this at an early stage isn’t clear. But one possibility is that mixed signals are particularly problematic in protein fingerprinting. That is to say, they can’t easily be classified as mixed. This could cause a significant fraction of proteins to be misclassified.
The Nautilus arraying approach works by creating a kind of adapter molecule which they call a SNAP (Structured Nucleic Acid Particle). This is a DNA nanoball (created using rolling circle amplification, similar to MGI in their sequencing platform). But it’s structured such that there’s a single site on the nanoball to which a protein can attach. The advantage here is that a SNAP can be relatively large and sit on a lithographically fabricated site on a surface, likely of a few 100nm. The result is an array of easily separable sites on a surface, each of which presents a single protein attachment site.
Their patents suggest a number of methods for making SNAPs or similar structures. But the DNA nanoball approach seems like the most obvious and the only one which appears to have experimental support. It looks like they need to do size selection on the nanoballs, which complicates the process somewhat. But they seem to have some images showing single dyes attached to the nanoballs.
How well this might work in a complete platform, with a complex sample is unclear.
Identifying/Fingerprinting Proteins
To me the patents relating to this part of the Nautilus approach felt the weakest. Essentially they say use a number of different affinity reagents (aptamers, or antibodies) to generate binding signals. Then use those binding signals to determine which protein is present.
So, you’d flow in one reagent, get a binding signal, flow in a second, get another signal, etc. All this binding information is then compared to a database of known protein binding fingerprints. The patent seems to refer to between 50 and 1000 reagents. In a youtube interview, Sujal suggested this generates 10 to 20Tb of image data.
They also suggest that you can also use affinity reagents that specifically bind to trimers or some other short motif. This almost then beings to look similar to a sequencing-by-hybridisation approach. Where you generate short reads and overlap them to recover the original sequence.
The patent I’ve looked at is completely theoretical, all the examples are simulations. The idea itself seems relatively obvious. The claims and specification make a big deal out of the process being iterative. But this doesn’t seem to be hugely significant to me, and somewhat obvious. The patent has a single claim. This claim in framed in terms of comparing binding measurements against a database. This suggests to me that they’re not seriously looking at sequencing-like applications.
Developing Libraries of Affinity Reagents
A third patent refers to methods of developing affinity reagents for use in the above process. Here they talk about methods for generating aptamers and other affinity reagents. The aptamer generation process seems to be a relatively standard aptamer evolution approach:
They seem to have performed a slightly smarter aptamer selection process than that described in the flowchart above. In this process they create candidate aptamers then sequence them on an Illumina sequencer. This gives them the sequence and location on the flowcell of each aptamer. They then wash a fluorescently labelled protein over the flowcell and measure binding. This gives them a high throughput way of measuring aptamer-protein binding efficiency. I suspect they’re not the first to do this however. The approach will likely mostly be complicated by the fact that Illumina have made it harder to modify the sequencing protocol on recent instruments.
They present some data from this approach, and show binding versus protein concentration for a few aptamers:
However, the aptamers they’ve discovered don’t seem to be covered in the patent. Perhaps there’s an unpublished filling which covers specific aptamers or other affinity reagents in more detail.
Elsewhere in the patent they discuss generating affinity reagents (likely antibodies) that specifically bind to 5mers. They propose doing this by first creating 2mer and 3mer specific affinity reagents and combining them.
The aptamer stuff in this patent is the most convincing. And I suspect they’re working on an aptamer based solution, however aptamers have a somewhat troubled history. It seems by no means easy to get an aptamer based platform working well. While the patent is interesting because it discloses some details of their approach. The patent itself doesn’t seem very strong. It originally had 131 claims. 111 of these have been cancelled, leaving a single independent claim.
Conclusion
In summary, they seem to be building an optical single molecule protein fingerprinting platform. Proteins are arrayed on a surface, exposed to a number (perhaps 100s) of fluorescently labeled affinity reagents. These are probably a combination of aptamers or antibodies.
By combining the binding information from all these different reagents, they can produce a unique fingerprint for a single protein. And by comparing this to a database of known proteins they can calculate the abundance of each protein in a sample. Because they’re using an optical approach this should be relatively high throughput. They also have IP on a chip based (QuantumSi/PacBio-like) platform, but to me this seems less scalable…
There are a number of applications for such a platform, but they mostly talk about Pharma (drug design, evaluation). Where such an approach would provide a more sensitive method of evaluating the performance of a drug, and how it effects protein expression.
For me, the most developed part of the approach is the arraying technology (using SNAPs). But this isn’t really required to get the platform up and running. It also doesn’t create any kind of IP barrier. It helps push throughput, but it isn’t clear to me that it’s of fundamental importance in building a protein fingerprinting platform.
The other parts of the approach (from the published patents) seem less well developed. I’d also note that while Sujal draws parallels with DNA sequencing, this approach is closer qPCR or DNA microarrays. Where you’re comparing detection events against a known database.
I’ll be watching with interest, but at the moment I’m more excited about approaches that provide “de novo” information that’s a little closer to sequencing than fingerprinting.